Big data is big.
And that was true even before the explosion of GenAI. In fact, with GenAI poised to generate a whopping 10% of the world’s data by 2025, the relationship between big data and AI has become even more interconnected.
Since the arrival of GenAI, many have reported that expenses associated with running their big data projects are becoming unsustainable. In June, SQream surveyed several hundred enterprises for its State of Big Data Analytics Report and found that 92% of companies surveyed are actively aiming to reduce cloud spend on analytics.
For enterprises there isn’t one right solution to grapple with these costs associated with the growth of data, with the market introducing an array of big data products and services. The number of options makes it hard for organizations to find the right tools, which can lead to higher costs and suboptimal performance.
Data acceleration platform SQream (article’s featured photo) recently participated in the TPC Express Big Bench tests to test the performance of its data lakehouse solution, SQream Blue.
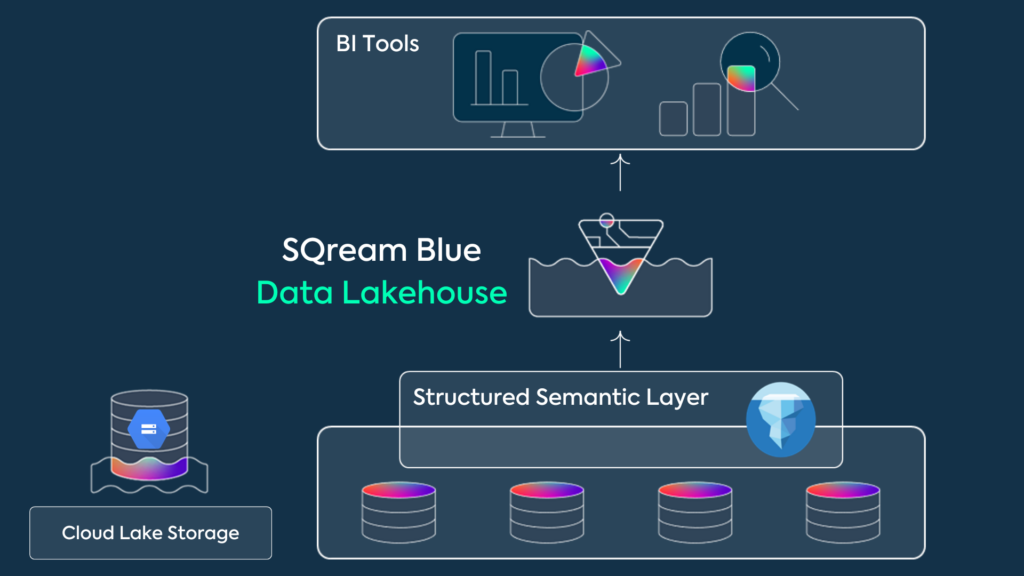
The results of the test exceeded benchmarks on several fronts, with the solution able to handle 30TB of data three times faster than Databricks’ Spark-based Photon SQL engine, and at 1/3 the cost.
The TPCx-BB testing system provides insight into the capabilities of big data solutions. Scenarios with set parameters provided a benchmark for organizations to better understand which solutions are likely to perform the most effectively for their data goals.
SQream Blue’s total runtime was 2462.6 seconds, with the total cost for processing the data end-to-end being $26.94. Databricks’ total runtime was 8332.4 seconds, at a cost of $76.94.
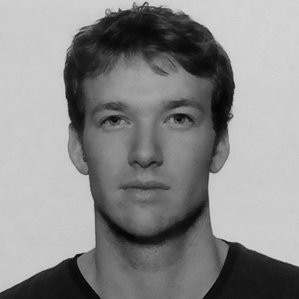
Said Matan Libis, VP Product at SQream, “In cloud analytics, cost performance is the only factor that matters. SQream Blue’s proprietary complex engineering algorithms offer unparalleled capabilities, making it the top choice for heavy workloads when analyzing structured data.”
“Databricks users and analytics vendors can easily add SQream to their existing data stack, offload costly intensive data and AI preparation workloads to SQream, and reduce cost while improving time to insights,” added Libis.
With enterprises surveyed in the above report typically handles over 1PB of data, such efficiency gains are set to translate into a huge reduction in costs.
For the benchmark, to test the capabilities of SQream at scale this was run on Amazon Web Services (AWS) with a dataset of 30 TB.
Generated data was stored as Apache Parquet files on Amazon Simple Storage Service (Amazon S3), and the queries were processed without pre-loading into a database.